FTC Tech Summit on AI
I finally had time to watch the AI tech summit very well-organised by the US Federal Trade Commission on 25 January. You can watch the full 4½ hour video here. I’ve tried to note some key points from each speaker below.
FTC CTO Stephanie Nguyen: FTC Office of Technology now has 12 technologists working on cases/investigations and engaging on policy and horizon scanning research.
Today’s summit is to understand competition in the AI tech stack: hardware/infrastructure, data and models, and consumer applications.
FTC Chair Lina Khan: We’ve seen how these AI tools can turbocharge fraud, entrench discrimination and enhance surveillance.
Will this be a moment of opening up markets and unleashing technologies, or will a handful of dominant firms lock up these possibilities for good?
When you concentrate production, you concentrate risk, as we see today with Boeing and many other large corporations whose market power masked decline of internal capacity.
With Web 2.0 aggressive strategies have solidified platform dominance while locking in business models damaging to privacy, journalism and children’s mental health.
FTC inquiry is launching inquiries into investments/partnerships by large AI firms, eg Microsoft/OpenAI.
Model training is emerging as a feature that could incentivise surveillance but cannot come at the expense of customers’ privacy and security.
Privacy violations are fuelling market power, enabling firms in turn to violate consumer protection laws.
We are focused on aligning liability with ability and control, eg robocall investigation looking upstream to VoIP providers.
Remedies should address incentives and establish bright line rules on data, eg face recognition and location data.
FTC workshop and report on creative works in generative AI lays out guardrails on protecting fair competition.
Panel 1: AI & Chips and Cloud
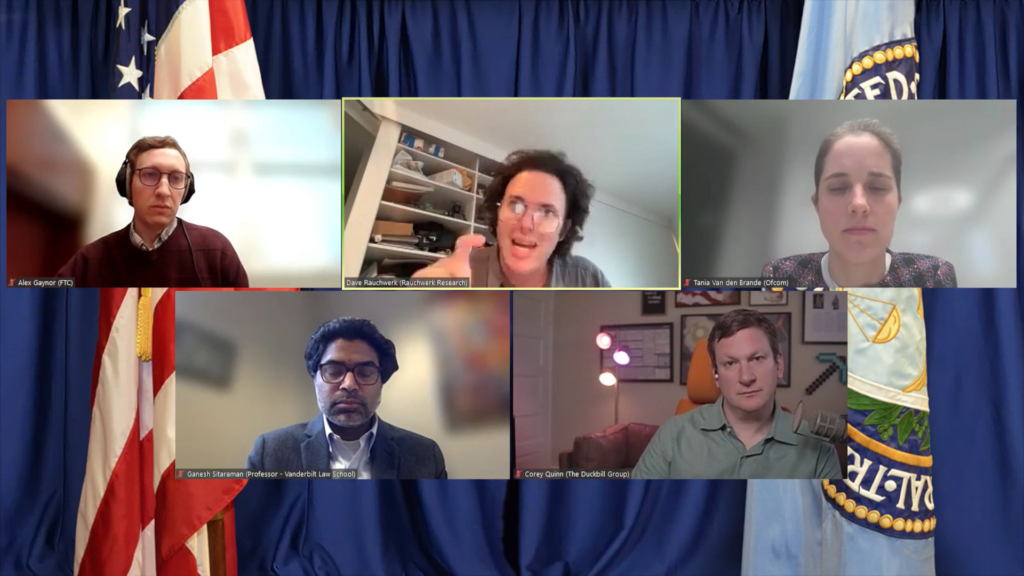
Tania Van den Brande, Ofcom Director of Economics: UK cloud is very concentrated towards AWS and Microsoft, and customers are struggling to switch, given egress fees and difficulty of reengineering for multiple cloud infrastructures and moving gradually. Discounting structures are problematic, discouraging multiple cloud usage. CMA is now conducting cloud market inquiry and will include AI in that.
Dave Rauchwerk, former semiconductor founder and tech entrepreneur: semiconductor startups are competing against hyperscalers, which are now building their own chips — MS, Amazon, Tesla — this is a further barrier to entry, alongside access to capital (competing with largest companies in world), which can maintain surveillance of innovation.
Very close partnership required for success with larger firms (Nvidia has worked closely with TSMC since the 1990s). About 5000 VCs are investing in AI startups, but only 300 in chip companies.
Dominant cloud firms are becoming a monopsony for the AI semiconductor firms, which could limit innovation in the functionality exposed to applications over time.
Real innovation and specialisation is possible at the chip layer, but the cloud companies aren’t buying them.
Intel is two companies — chip designer, and chip manufacturer in “fabs”/foundries. Because it’s vertically integrated it doesn’t have the incentive to help rival chip designers, and can monitor what they’re doing. The US needs a national pure play foundry, like TSMC.
Prof Ganesh Sitaraman, Vanderbilt Law School: AI tech stack: app -> model -> cloud -> chips. Lower layers show increased concentration (Nvidia, TSMC, ASML) — at chip layer, with national security concerns (Taiwan). Firms can preference their own vertically integrated business lines, discriminate between customers, and raise prices/decrease quality. And this can deter smaller firms who don’t have the ability to deploy innovative apps across a global ecosystem. Hyperscalers can copy innovations and give them preferential treatment.
If governments get too dependent on large firms they can become “too big to prosecute” (like too-big-to-fail banks earlier this century.)
Potential solutions: structural separation, to prevent self-preferencing and other harms from vertical integration; non-discrimination rules, on prices, T&Cs, self-preferencing; transparency on T&Cs; interoperability rules.
Cory Quinn, chief cloud economist at The Duckbill Group: People tend to miss how much work it is to train large models. Amazon just spent $65m on one training. Amazon make its own chips but are using Nvidia GPUs which cost $30k. Nobody knows how Nvidia are allocating their limited supply but it certainly helps when customers have deep historical links with the firm.
Market has now tipped and the centralisation risk to resilience is massive.
These cloud companies already use the language of monopolists. The cost of entry to large-scale AI is already massive.
Egress fees as such are not objectionably large. The problem is firms want to move large quantities of data to compute facilities, and large egress fees obstruct that.
We have an Nvidia monoculture now. They are the major bottleneck, followed closely by their cloud customers. We should treat them all like utilities. In the short term more transparency over GPU distribution would help.
It’s extremely difficult and time-consuming for firms to move from one cloud provider to another.
FTC Commissioner Rebecca Slaughter: We are still dealing with the fall-out from the relaxed regulatory approach to the era of Big Data, adtech and social media/commercial surveillance. Despite early warnings about privacy and consolidation, regulators and legislators targeted only the most egregious conduct at first. And now markets have consolidated into extraordinarily large companies, the once vibrant US arts and journalism sectors are in crisis, while disinformation and material damaging teen health has proliferated. We have the knowledge and experience to see the AI era play out differently.
FTC is studying whether AI investments lead to a heavily concentrated market, while avoiding merger inquiries.
Consumer protection rules are also important. Honest marketing claims are deeply pro-competitive.
AI models can use consumer data in ways that entrench inequalities and access to opportunities.
Panel 2: AI & Data and Models
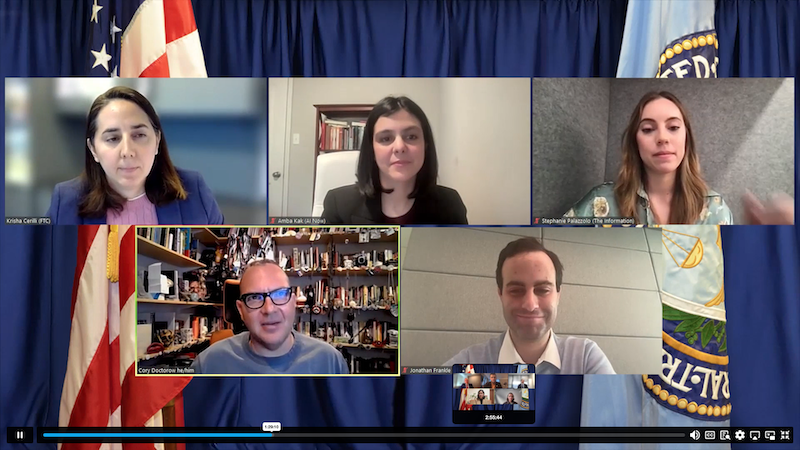
Cory Doctorow, sci-fi author and EFF Special Adviser: copyright law is not a great framework for dealing with AI data issues. It neglects the structure of many creative industries: monopsonies (5 publishers, 3 labels, 2 adtech firms). Giving artists more money won’t work in this condition as it will be taken by employers. Instead we need labour and privacy law.
AI investors are being pitched on automation and reducing headcount, not an augmenting services and human capabilities.
EFF talks about Privacy First, a potential coalition for a federal privacy law with a private right of action, much broader than AI. But specifically we’ve seen AI systems memorising then regurgitating highly personal information. Privacy law would provide many remedies for AI problems.
We need to think about data beyond a property regime but rather how to avoid harms to stakeholders, eg displace creative workers, produce grotesque privacy invasions such as non-consensual pornography, mine people’s data to make inferences adverse to their interests. We describe the most valuable things in the world — people — without property language.
Jonathan Frankel, chief data scientist at DataBricks: there is huge diversity in AI business models, from OpenAI/Anthropic/Cohere/Midjourney/Adobe access to hugely expensive models, to helping firms train open source models on their own data. Frankel’s experience in dealing with many input firms such as cloud is the markets are incredibly competitive.
Competition is not sufficient to have good outcomes. We are rapidly moving from an open, research-like approach to corporate R&D to a closed, “competitive intelligence” situation. There is a lot of regulatory/legal uncertainty so there is an incentive for firms to be secretive about what data they are using to reduce risks. There is strong pressure to get to market, which makes it harder to get things right. Data curating is one of the biggest costs for training.
“Open source” term brings baggage. Better: access to models, and transparency. Do you have access to model weights, to manipulate it, work with it yourself — like Llama but not GPT-4. Do you know how the model was built? What data was used to train it, details of hyperparameters — not true of Llama 2. Both = open source.
Making models freely available in this way has pros/cons. Pro: nobody mediates access to model. You can fully customise it, whether as a hobbyist, researcher, or large firm. You can build on the (very expensive) work of others. Great for science. You control your own fate, models won’t change under you. You can serve it yourself and know all the inputs. These are benefits of access.
Transparency part is more complex. Firms so far have been generous — is this sustainable?
Also has consequences for startups — discourages particular types of investments? Much more complex: the risks of giving people control over an artefact. Finally: this is not binary, it’s a whole design space.
We need centralised, shared, publicly funded resources for improving AI safety.
Amba Kak, Executive Director at AI Now Institute: data quality (high levels of curation, feedback, niche datasets like healthcare and finance, assurances of accuracy and diversity) and scale are acting as barriers to entry. Big Tech firms have a big advantage from the last decades of commercial surveillance, and near-unlimited capital to invest to make datasets more robust. Will these port to the so-called AI startups they are investing in? How will large tech firms leverage their relationships with publishers and the media to maximise access and exclusivity? This isn’t unique to foundation models; getting data for fine-tuning models is also becoming more difficult. These data advantages are very self-reinforcing. Sam Altman has said “personalised” access will be the next phase of OpenAI, while giving the firm a huge advantage over competitors in access to data.
Data minimisation is the key principle, more important than ever in the age of AI, not less.
Even today’s open source firms are operating in a highly concentrated market where they benefit from network effects. SMEs will need the same protections against eg self-preferencing as closed source users.
Huge danger that AI and innovation is perceived to require lax privacy rules. The opposite is true. Data minimisation isn’t new — the lesson from the GDPR is not allowing too much room for interpretation (eg is behavioural advertising a legitimate business purpose?)
Scale/speed as proxies for progress are too limited. What about eg impact on the environment? Who decides/shapes what counts as innovation for the public good? One way forward is to go back to the drawing board and have a much more broad-ranging conversation dominated by public not narrowly private interests, rather than be passive recipients/subjects of the tech trajectory.
Stephanie Palazzolo, The Information: new group of startups trying to build non-transformer models (transformer models such as GPT and Claude).
Getting funding: investors looking at talent (eg former Google, top US colleges), whether startups are competing with OpenAI (even potentially, eg following OpenAI’s developer day announcements), how close they are to market. Capital depends on whether you can strike deals for valuable data, pay for chips… Early stage investors care about growth; later-stage care about cash generation and margins. Much harder for startups to generate cash due to entry costs.
Sustainability of open source models is important for startups. Difficult to imagine open source developers and users can compete against the bleeding-edge, largest models from Google/OpenAI/Anthropic. And we need a lot more funding of academic labs, whether on data or chips. Compared number of GPUs Meta is buying vs Carnegie-Mellon University’s leading lab — huge difference.
FTC Commissioner Alvaro Bedoya: We shouldn’t let all the media hype and attention being paid to generative AI/LLMs distract us from the fact that other forms of automated decision making are today having a much bigger impact on people’s lives. Focuses his remarks on bias in these latter systems, and the FTC’s Rite Aid case on biased facial recognition.
We need to appreciate what’s at stake. These systems affect our basic ability to live our lives with dignity, with fairness, to get the healthcare we need, apartments we rent, jobs we apply for…
Algorithms are not an excuse. Firms need to ask hard questions about how systems work, how they affect people they are used against…
Success would look like people controlling technology, not the other way around. People feeling in control of tech, knowing when it’s being used to make decisions about them, why those decisions were made, knowing their remedies. And competition-wise, we use tech which proves itself the best in the marketplace on its merits — products that work, which people like, not just because it’s put out by a $1tn company.
Panel 3: AI & Consumer Applications
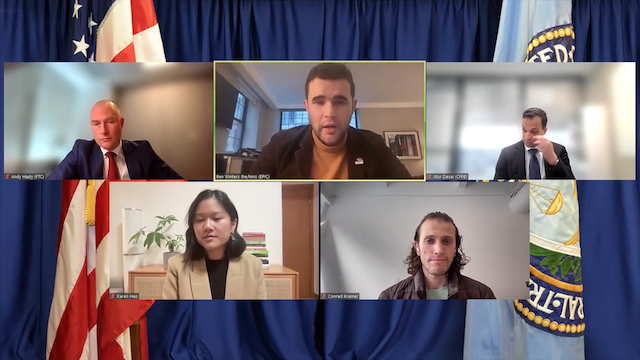
Conrad Kramer, AI startup: primarily startups need access to models to build products. Consumers typically interact with a product which embeds a model. Firms can access an existing service; train their own (needs lots of resources and expertise); or fine-tune an open model. Open source models are little bit lagging behind on quality. They are cheaper to acquire but still need compute resources for inferences and if needed fine-tuning/retraining. But they are usually completely transparent on data sources, which is useful in building a better product.
Model evaluation metrics are rudimental — standard questions and known answers. Needs a lot of qualitative understanding. It’s really hard. Correctness is domain-dependent, text generated for communications needs attention to precision, accuracy as well as tone.
We currently see an explosion of companies, some of which are training models for a fee. Or download the weights for a model for free from Hugging Face and run it on your own computer, but this needs a powerful machine — so better to run it on a cloud server and run it own behalf of users. There are providers of this service, which are competing on compute cost.
Excited for the potential to improve people’s lives, eg auto-filling forms and other rote/repetitive tasks to let people focus on more human activities. Is concerned about privacy, people’s control over their data.
Some products give users meaningful privacy controls (like iPhone microphone access to apps). Best systems keep data close to you, ideally on your own system, and when/how it’s used.
It is possible to build AI products which obey the law, protect users’ privacy, are safe — in terms of current harms, not x-risk. Thinks startups which innovate on ways to provide better privacy and safety will ultimately succeed.
Karen Hao, journalist: consumers are excited to use these tools to unlock their creativity, like getting ideas from ChatGPT, or using StableDiffusion to generate concept work, like building plans or poster design. Parents want to engage with kids educationally, have an interactive storytime. But there is also a huge amount of risk, especially on lack of transparency: ambiguous/deceptive marketing, and obfuscation. LLMs have a problem with hallucinations but big providers paper over them, eg Nadella claiming they are just like better search, OpenAI partnering with legal assistive services. People often don’t even realise they are dealing with an AI-based system.
Original AI Safety definition by Anthropic co-founders is not related to privacy, security, fairness, economic impacts or military applications… it is about rogue AI, existential threats… but in the public domain, “safety” means something completely different. So firms are now using it in that way in their marketing, while still causing harm. (There was a NeurIPS conference panel on this last year). AI developers meanwhile are focusing their safety concerns on AGI. Hao recommends paper on Concrete Problems in AI safety, Revisited by Deborah Raji.
We need to really question what companies say, not only in terms of AI safety and marketing, but the ways they frame what is good for us. It doesn’t feel like we’re living in a democracy right now if a company just gets to decide… OpenAI launched ChatGPT almost on a whim, and now we’re living in this new era and have to grapple with that. I don’t feel like any of us had any democratic agency over that. We should be demanding more of these firms than retroactive excuses.
Ben Winters, Electronic Privacy Information Center: recently co-authored report on AI harms [Generating Harms: Generative AI’s Impact & Paths Forward]. Looked at social as well as individual harms. Sources of these harms: widespread availability of these chat tools; enabling harassment and impersonation; increasingly opaque data collection (thanks to lack of US federal privacy law); environmental impact; data security risk of all this data maximisation; labour manipulation, theft and displacement; discrimination such as entrenchment of discriminatory stereotypes; market power and concentration.
AI industry has a “man behind the curtain” problem, with needless overcomplicating. Focus on x-risk is just a distraction for legislators from current harms, while industry is not doing basic things like putting the transparency burden on the company not on the consumer to investigate something you cannot possibly understand if you spend half a day on it. Audits, impact assessments… but also the norm of respecting customers and valuing not just the expensive data you can buy from the NY Times, but everybody’s data.
Nothing is inevitable (that AI will be everywhere; that we face existential risk). This doesn’t have to be as complex as the largest companies want. We need to reassure consumers, regulators, legislators they can understand it and push back. And other laws do exist… civil rights, consumer protection, fair competition… we also need federal privacy law, and law which bans unconscionable uses of AI.
Atur Desai, US Consumer Finance Protection Bureau: CFPB is doing a lot related to AI. In reality, complex models have been used in consumer financial markets for a long time, eg in credit scoring. So a robust set of federal laws exist. Eg companies must provide accurate explanations of why they have denied a credit application (no matter how complex their model). CFPB has issued a notice for information to credit brokers. Doing a lot of work on capacity building internally, with a technologist programme embedding data scientists, ML experts etc. in enforcement teams.
Breaking the law should not be a company’s competitive advantage. We need ways to encourage whistleblowers where that is happening.
AI is an amorphous marketing term, describing sometimes very simple and sometimes very complex models. “Safe AI” is a murky mishmash of words. Deceptive marketing laws exist and CFPB is enforcing them already against algorithmic systems.
Henry Liu, Director of FTC Competition Bureau: Outsize market power can distort the path of innovation. FTC has already taken enforcement action such as against Broadcom and Nvidia to protection chip competition. FTC will have better powers to require firms to produce information.
Sam Levine, Director of FTC Consumer Protection Bureau: technologists are part of dozens of enforcement actions and really important for the work of the Commission. In engaging with AI we must learn from how we failed to fully deal with the Web 2.0 era. Privacy self-regulation was a serious error, and industry did not make privacy a priority. Bureau published an AI report in 2022 and guidance in 2023. We have now required algorithmic models trained on illegal acquired data be deleted. We have launched action against voice impersonation fraud. We have made clear firms cannot retain children’s data indefinitely, especially to train models; or use models that harm consumers. We are using every tool to protect the public from emerging harms.